Treating physicians need information about the molecular subtype of the tumor if they are to provide targeted therapy for colorectal carcinoma. A research team from University Hospital Zurich and the University of Oxford have now developed a method to predict the molecular classification of colorectal cancer from digital pathology slides.
Colorectal cancer is the third most common malignant tumor in men and women with approximately 1.8 million new cases globally per year, including around 4,000 in Switzerland. Surgery, radiation and chemotherapy as well as precision therapeutics are the established treatment options, but are associated with relevant side effects. Precise information about the molecular subtype of the tumor using RNA sequencing can support patient stratification for personalized therapy. Yet cancer classification through RNA sequencing remains a resource-intensive, costly process: examining a single sample costs over CHF 1,000. Further, up to 20 percent of samples cannot be conclusively classified due to insufficient availability of material or ambiguous results.
Research advances through image analysis and artificial intelligence
A research team led by Prof. Viktor Kölzer, Institute of Pathology and Molecular Pathology at University Hospital Zurich (UHZ), and Prof. Jens Rittscher, Institute of Biomedical Engineering at the University of Oxford, have now developed a much cheaper, faster method: they use artificial intelligence to analyze high-resolution images of histological slides. This allows the subclassification of colorectal tumors into one of four distinct transcriptional subtypes and gives an indication of optimal treatment strategies. Unlike RNA sequencing, which has been the gold standard thus far, this purely image-based procedure does not require any additional tissue material. It works even with very small tissue fragments and makes it possible to classify tissue samples that were previously inaccessible due to the technical limitations. The procedure also has the potential to lower costs considerably. Image-based procedures could therefore potentially revolutionize personalized therapy in colorectal cancer. Yet in order to use the new technology, the histological slides need to be appropriately prepared: “to use artificial intelligence for tumor analysis in daily diagnostic practice, we need to digitize pathology workflows,” says Prof. Kölzer.
Strategic importance for personalized medicine
In April this year, Prof. Kölzer accepted the post of Assistant Professor in Digital Pathology at UHZ. This professorship is the first of its kind in Switzerland with strategic importance for personalized medicine. Prof. Kölzer initialized this project on AI-supported cancer classification during his time at the University of Oxford, in a strong interdisciplinary collaboration with the pathologists, bioinformaticians, clinicians and statisticians of the multi-institutional Stratification in COloRecTal Cancer (S-CORT) Consortium. The study involved the analysis of 1,553 digital tissue slides with data on RNA expression, gene mutations and clinical progression using the latest machine vision and artificial intelligence technologies. This new technology was first published as a preprint in late May 2019 and is recommended for validation in prospective, randomized clinical trials. According to Prof. Kölzer, “After validation, we will be able to centralize the classification of colorectal tumors and release the technology for use.” Scans of histological slides could be sent to university centers, where they would be evaluated and the results returned electronically. In the long term, the method could also be used for other tumor types or even other diseases. Prof. Maughan, leader of the S:CORT consortium comments: “this research shows that, with the help of computer analysis, it is possible to detect complex biological patterns from the way the cancer looks under the microscope using routine ways to prepare tissue slides. This has great potential for providing information on how the cancer will behave in the individual and use this in the future to guide treatment decisions”.
For a preprint of the study, see https://doi.org/10.1101/645143
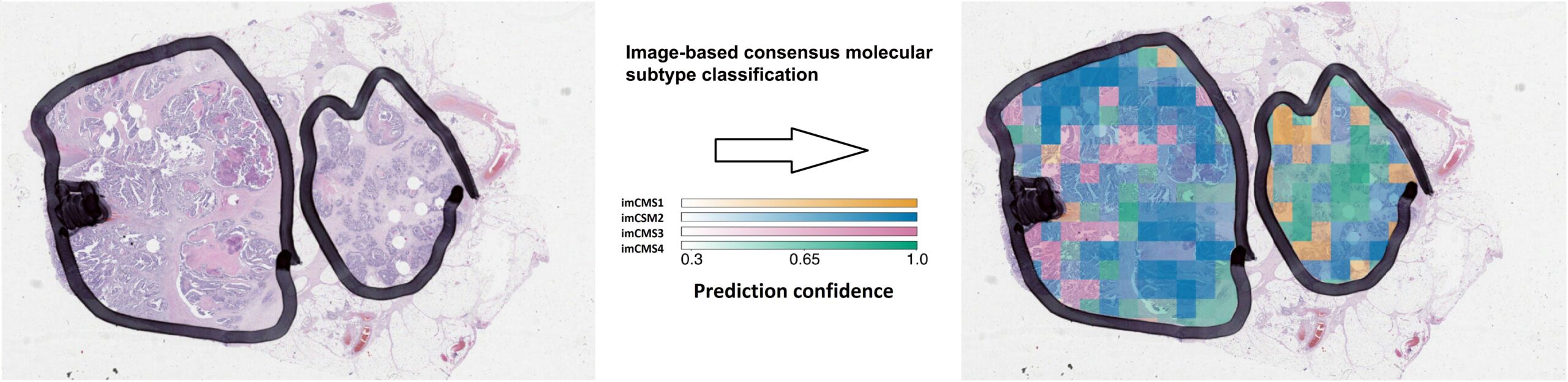
The image-based consensus molecular subtypes (imCMS) model can be used to predict the molecular classification of each individual image region in patient tumor samples. This process takes just a few minutes and requires no further patient material.